Ghizlane Echbarthi
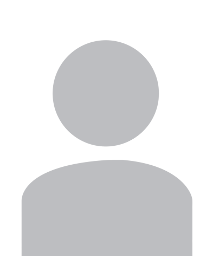
Ancien membre LIRIS depuis le : 2018-10-01
Qualité (LIRIS) | Doctorant |
Équipe(s) | GOAL |
Employeur | Université Claude Bernard Lyon 1 |
Thèse | Techniques de graphes pour l’agrégation de données (cliquer pour voir les détails) |
Publications (IdHAL : ghizlaneechbarthi)
Publications LIRIS pour Ghizlane Echbarthi (2)
- 2016 (1)
- Conférences (1)
- Conférences internationales avec comité de lecture (1)
- Ghizlane Echbarthi & Hamamache Kheddouci (2016). « Streaming METIS Partitioning ». ASONAM 2016, SAN FRANSISCO (États-Unis). HAL : hal-01405170.
- 2014 (1)
- Conférences (1)
- Conférences internationales avec comité de lecture (1)
- Ghizlane Echbarthi & Hamamache Kheddouci (2014). « Fractional greedy and partial restreaming partitioning: New methods for massive graph partitioning. ». Big Data Conference, Washington DC (États-Unis). HAL : hal-01282071.
.