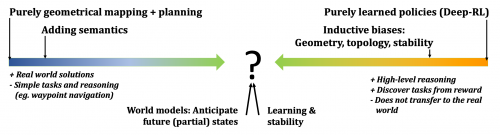
The chair position in Artificial Intelligence "REMEMBER", proposed by a LIRIS researcher, has been selected by an international committee
The AI chair position “Remember: Learning Reasoning, Memory and Behavior”, led by Christian Wolf and involving Laetitia Matignon (LIRIS) and Olivier Simonin (CITI, Inria) and Jilles Dibangoye (CITI, Inria) will study the connections between machine learning, optimal control and mobile robotics. It is funded by the French Agency Agence National de Recherche (ANR), by the company Naver Labs Europe and by INSA de Lyon.
The last years have witnessed the soaring of Machine Learning, which has provided disruptive performance gains in several fields. Apart from undeniable advances in methodology, these gains are often attributed to massive amounts of training data and computing power, which led to breakthroughs in speech recognition, computer vision and language processing. In this challenging project, we propose to extend these advances to sequential decision making of agents for planning and control in complex 3D environments.
In this chair project, we will focus on methodological contributions (models and algorithms) for training virtual and real agents to learn to solve complex tasks autonomously. In particular, intelligent agents require high-level reasoning capabilities, situation awareness, and the capacity of robustly taking the right decisions at the right moments.
We argue, that learning the behavior policies crucially depends on the algorithm's capacity of learning compact, spatially structured and semantically meaningful memory representations, which are able to capture short-range and long-range regularities in the task and the environment. A second key requirement is the ability to learn these representations with a minimal amount of human interventions and annotations, as the manual design of complex representations is up to impossible.
The research project aims to address these issues with four axes: (i) adding structure and priors to Deep-RL algorithms allowing them to discover semantic and spatial representations with metric and topological properties; (ii) learning situation aware models generalizing to real environments with geometry and self-supervised learning; (iii) learning world-models and high-level reasoning for mobile agents, and (iv) adding stability priors from control-theory to Deep-RL.
The planned methodological advances of this project will be continuously evaluated on challenging applications, partially in simulated environments, partially in real environments with physical robots in large scale scenarios.