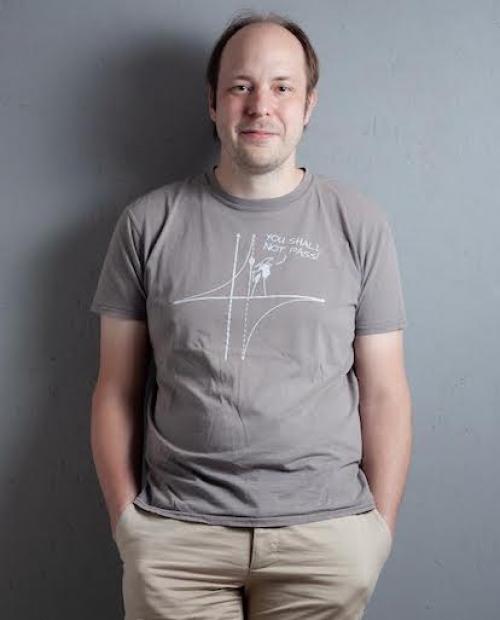
LIRIS Seminar - Sébastien Destercke - Learning in an imperfect world, with a focus on uncertain data.
Accounting for uncertainties to obtain better or more robust predictive models is a common task in machine learning. While such tasks are most often seen from a purely probabilistic perspective, it can be interesting to mix it with a hint of imprecision, for instance to provide robust inferences and/or allow for a richer modelling of uncertainties. In this talk, I will browse different situations where such imprecision can be embedded in standard learning frameworks, focusing in peculiar on the case of imprecise and imperfect data, and on those situations where accounting for imprecision can actually help the learning process rather than representing a burden for it.
Short vita: Sébastien Destercke's research mostly concerns the representation and treatment of information under severe uncertainty, i.e., when data and information are scarce, unreliable, imprecise, etc.
Sébastien is currently focusing his research on problems related to preference learning, risk/reliability analysis and signal treatment/image analysis.
He also give courses (for professionals and students) concerning these topics.
Specialties: Uncertainty modeling and treatment: information fusion, decision making, information representation, uncertainty propagation, model validation, supervised learning, risk analysis. He is the holder of the SAFE AI Chair, mainly funded by UTC fondation pour l'innovation, and co-funded by the Sorbonne Center for Artificial Intelligence (SCAI).