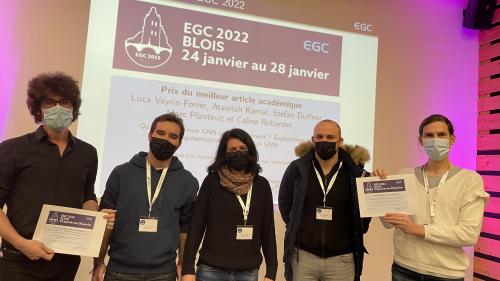
Best academic paper award and best application paper award at EGC 2022
Papers "Qu’est-ce que mon GNN capture vraiment ? Exploration des représentations internes d’un GNN" presented by Luca Veyrin-Forrer (LIRIS, DM2L) and "Classification automatique d'articles encyclopédiques" presented by Alice Brenon (LIRIS, DM2L/TWEAK & ICAR), have won the best academic paper and the best application paper awards, respectively at the EGC 2022 conference.
Best academic paper award:
Qu’est-ce que mon GNN capture vraiment ? Exploration des représentations internes d’un GNN
Luca Veyrin-Forrer, Ataollah Kamal, Stefan Duffner, Marc Plantevit and Celine Robardet
Abstract:
While existing GNN’s explanation methods explain the decision by studying the output layer, we propose a method that analyzes the hidden layers to identify the neurons that are co-activated for a class. We associate to them a graph.
Paper : https://editions-rnti.fr/?inprocid=1002725
--
Best application paper award:
Classification automatique d'articles encyclopédiques
Ludovic Moncla, Khaled Chabane, Alice Brenon
Ludovic Moncla, Khaled Chabane, Alice Brenon
Abstract:
This article proposes a comparative study of different supervised classification approaches applied to the automatic classification of encyclopaedic articles. Our training corpus is com- posed of the 17 volumes of text of the Encyclopédie by Diderot and d’Alembert (1751-1772) representing a total of about 70,000 articles. We have experimented different approaches for text vectorization (bag of words and word embeddings) combined with classical machine learn- ing methods, deep learning and BERT architectures. In addition to the comparison of these different approaches, our objective is to automatically identify the domains of the unclassified articles of the Encyclopaedia (about 2400 articles). The best model obtains 89% of average f1-score for the 38 classes. Moreover, our study highlights the difficulty of classifying certain semantically close classes. All the code developed and the results obtained in the framework of this project are available in open-source.
This article proposes a comparative study of different supervised classification approaches applied to the automatic classification of encyclopaedic articles. Our training corpus is com- posed of the 17 volumes of text of the Encyclopédie by Diderot and d’Alembert (1751-1772) representing a total of about 70,000 articles. We have experimented different approaches for text vectorization (bag of words and word embeddings) combined with classical machine learn- ing methods, deep learning and BERT architectures. In addition to the comparison of these different approaches, our objective is to automatically identify the domains of the unclassified articles of the Encyclopaedia (about 2400 articles). The best model obtains 89% of average f1-score for the 38 classes. Moreover, our study highlights the difficulty of classifying certain semantically close classes. All the code developed and the results obtained in the framework of this project are available in open-source.
Paper : https://editions-rnti.fr/?inprocid=1002717
--
Conference website: https://egc2022.univ-tours.fr