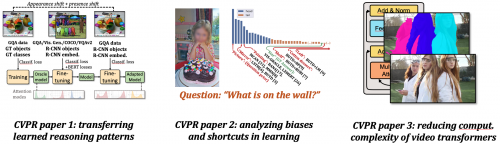
3 papers accepted at the conference CVPR by groups IMAGINE and SICAL
The work of the team was done by PhD students Corentin Kervadec (2 Papers), who is currently doing an industrial thesis with Orange and co-supervised by C. Wolf on the LIRIS side, and G. Antipov and M. Baccouche from Orange. One of the papers is a collaboration with the SICAL team (PhD student Théo Jaunet and co-supervisor R. Vuillemot).
One paper is a collaboration with University of Guelph, Canada, with first author PhD student B. Duke, enrolled in UoG, and supervisor Graham W. Taylor.
All three papers are based on large scale learning of attention-based neural networks, nicknamed “transformers” by the community.
CVPR Paper #1
Corentin Kervadec, Théo Jaunet, Grigory Antipov, Moez Baccouche, Romain Vuillemot and Christian Wolf. How Transferrable are Reasoning Patterns in VQA? To appear in International Conference on Computer Vision and Pattern Recognition (CVPR), 2021. [Openreview Link]
This paper explores vision and language learning and how learned patterns of reasoning can be transferred between models trained on different datasets. A visual data analytics tool was developed and is available online: https://reasoningpatterns.github.io
CVPR Paper #2
Corentin Kervadec, Grigory Antipov, Moez Baccouche and Christian Wolf. Roses Are Red, Violets Are Blue... but Should VQA Expect Them To? To appear in International Conference on Computer Vision and Pattern Recognition (CVPR), 2021. [Arxiv Link]
This paper explores the biases exploited by state-of-the-art neural models in vision and language learning. In a large-scale study involving 7 VQA models and 3 bias reduction techniques, we also experimentally demonstrate that these models fail to address questions involving infrequent concepts; we create a new benchmark and dataset addressing these issues and we provide recommendations for future directions of research.
CVPR Paper #3
Brendan Duke, Abdalla Ahmed, Christian Wolf, Parham Aarabi and Graham W. Taylor. SSTVOS: Sparse Spatiotemporal Transformers for Video Object Segmentation. To appear in International Conference on Computer Vision and Pattern Recognition (CVPR), 2021 (oral presentation). [Arxiv Link]
This paper introduces a Transformer-based approach to video object segmentation with a particular focus on reducing computational complexity of the classical quadratic cost of self-attention.