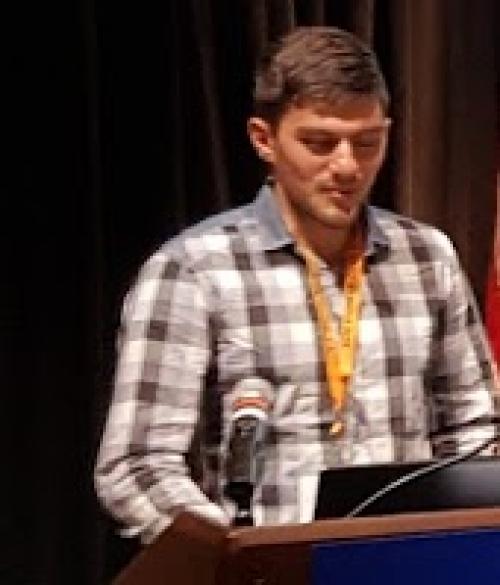
Vlad Nitu, winner of the IMPULSION 2020 call for projects
The number of edge devices and the data produced by them has grown tremendously over the last 10 years. While in 2009 mobile phones only generated 0.7% of worldwide data traffic, in 2018 this number exceeded 50%. In addition, user’s privacy is an important issue since privacy-related scandals (e.g. PRISM or Cambridge Analytica) continue to unfold, and new regulations come into force (e.g. the EU’s General Data Protection Regulation). Therefore, large industrial players are now seeking to exploit the rising power of edge devices to reduce the demand on their server infrastructures while protecting their users’ privacy. In this context, a new computing paradigm has emerged, spearheaded by big tech companies (especially Google) as Federated Learning. The latter offloads the cloud storage and computation costs of ML applications onto mobile devices by training a global model on decentralized data stored locally. Even if Federated Learning opens wonderful perspectives in privacy-sensitive domains (e.g., healthcare) so far reluctant toward machine learning, it unveils a new set of challenges related to system abstractions, energy efficiency and fault tolerance.