Lucas Foulon
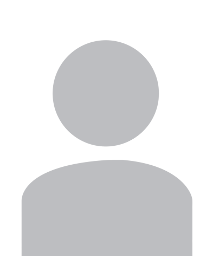
Ancien membre LIRIS depuis le : 2021-06-30
Qualité (LIRIS) | Doctorant |
Équipe(s) | DM2L |
Employeur | Autre |
Thèse | Détection d'anomalies dans les flux de données par structure d'indexation et approximation. Application à l'analyse en continu des flux de messages du système d'information de la SNCF (cliquer pour voir les détails) |
Publications (IdHAL : lucas-foulon)
Publications LIRIS pour Lucas Foulon (5)
- 2022 (1)
- Éditions scientifique d'ouvrages (livres, chapitres, colloques, congrès, n° spéciaux) (1)
- Lucas Foulon, Christophe Rigotti, Serge Fenet & Denis Jouvin (2022). « Anomaly Detection Based on Sequence Indexation and CFOF Score Approximation ». Advances in Knowledge Discovery and Management, Springer International Publishing, pp. 47-61. doi : 10.1007/978-3-030-90287-2_3. HAL : hal-03881940.
- 2020 (1)
- HDR, thèses (1)
- Thèses (1)
- Lucas Foulon (2020). « Détection d'anomalies dans les flux de données par structure d'indexation et approximation. Application à l'analyse en continu des flux de messages du système d'information de la SNCF ». HAL : tel-03089142.
.
- 2019 (3)
- Conférences (2)
- Conférences internationales avec comité de lecture (1)
- Lucas Foulon, Serge Fenet, Christophe Rigotti & Denis Jouvin (2019). « Scoring Message Stream Anomalies in Railway Communication Systems ». LMID 2019 - IEEE Workshop on Learning and Mining with Industrial Data, 11 novembre 2019, Beijing (Chine), pp. 1-8. doi : 10.1109/ICDMW.2019.00114. HAL : hal-02357924.
.
- Conférences nationales avec comité de lecture (1)
- Lucas Foulon, Christophe Rigotti, Serge Fenet & Denis Jouvin (2019). « Approximation du score CFOF de détection d’anomalie dans un arbre d’indexation iSAX : Application au contexte SI de la SNCF ». EGC 2019 - 19ème Conférence francophone sur l'Extraction et la Gestion des Connaissances, 25 janvier 2019, Metz (France), pp. 1-12. HAL : hal-02019035.
.
- Autres (1)
- Lucas Foulon, Serge Fenet, Christophe Rigotti & Denis Jouvin (2019). « Detecting Anomalies over Message Streams in Railway Communication Systems ». AALTD@ECML/PKDD 2019 - 4th Workshop on Advanced Analytics and Learning on Temporal Data. Poster, 20 septembre 2019, Wurzburg (Allemagne). Poster. HAL : hal-02357927.
.