Clément Peyrard
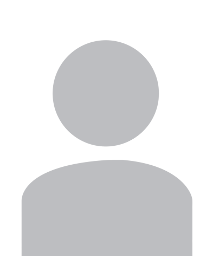
Ancien membre LIRIS depuis le : 2017-10-31
Qualité (LIRIS) | Doctorant |
Équipe(s) | Imagine |
Employeur | Institut National des Sciences Appliquées de Lyon |
Thèse | Les méthodes de super-résolution pour la classification et la reconnaissance d'objets dans les vidéos (cliquer pour voir les détails) |
Publications (IdHAL : clement-peyrard)
Publications LIRIS pour Clément Peyrard (3)
- 2016 (1)
- Conférences (1)
- Conférences internationales avec comité de lecture (1)
- Clément Peyrard, Christophe Garcia & Moez Baccouche (2016). « Blind Super-Resolution with Deep Convolutional Neural Networks ». ICANN 2016: 25th International Conference on Artificial Neural Networks, 9 septembre 2016, Barcelona (Espagne), pp. 161-169. doi : 10.1007/978-3-319-44781-0_20. HAL : hal-01421034.
- 2015 (2)
- Conférences (2)
- Conférences internationales avec comité de lecture (2)
- Clément Peyrard, Franck Mamalet & Christophe Garcia (2015). « A Comparison between Multi-Layer Perceptrons and Convolutional Neural Networks for Text Image Super-Resolution ». International Conference on Computer Vision Theory and Applications, 14 mars 2015, Berlin (Allemagne). doi : 10.5220/0005297200840091. HAL : hal-01260671.
- Clément Peyrard, Moez Baccouche, Franck Mamalet & Christophe Garcia (2015). « ICDAR2015 Competition on Text Image Super-Resolution ». 13th International Conference on Document Analysis and Recognition (ICDAR 2015), 26 août 2015, Tunis (Tunisie). HAL : hal-01157903.